The secret to a strong analytical culture is hiding in plain sight - an organization-wide obsession with attribution and segmentation.
In a prior post, I outlined evolutionary steps in a strong analytics practice. The first step, tracking metrics, is table stakes. But, when metrics are contextualized with a rich understanding of its distributions and segments, the latent power in the data can be unlocked.
As an example, measuring user retention for an organization is the first step - but building the organizational muscle to analyze retention variations across segments across time, based on the user’s acquisition source, their product usage, their operational experience, their sensitivity to price and promotions, their demographics, and their qualitative feedback data - these will provide directly actionable tactics or help shape product strategy to improve retention. It’s not the “what” but the “why” or the “how” that makes data actionable.
Now, how do you build and nurture this organizational muscle? Today, metric segmentation is baked into pre-computed datasets behind metric dashboards. Most organizations pull in a small static set of entity attributes like some demographic info or a sales or marketing channel, and use that to define segments to cut specific reports. On the other end of the spectrum, some orgs, eager to be “data-driven”, create such a sprawl of disconnected reports and incoherent segment cuts that it becomes nearly impossible to isolate signal from noise.
To address this from a tooling standpoint, we can leverage the power of a business semantic graph - an abstraction that the data and business teams can coherently collaborate on. In prior posts, I have shown examples of such graphs, and discussed how it represents a computational graph showcasing what’s analytically possible. In the first graph below, you can see that the metric sum_leads has seven attributes to segment and cut the metric.
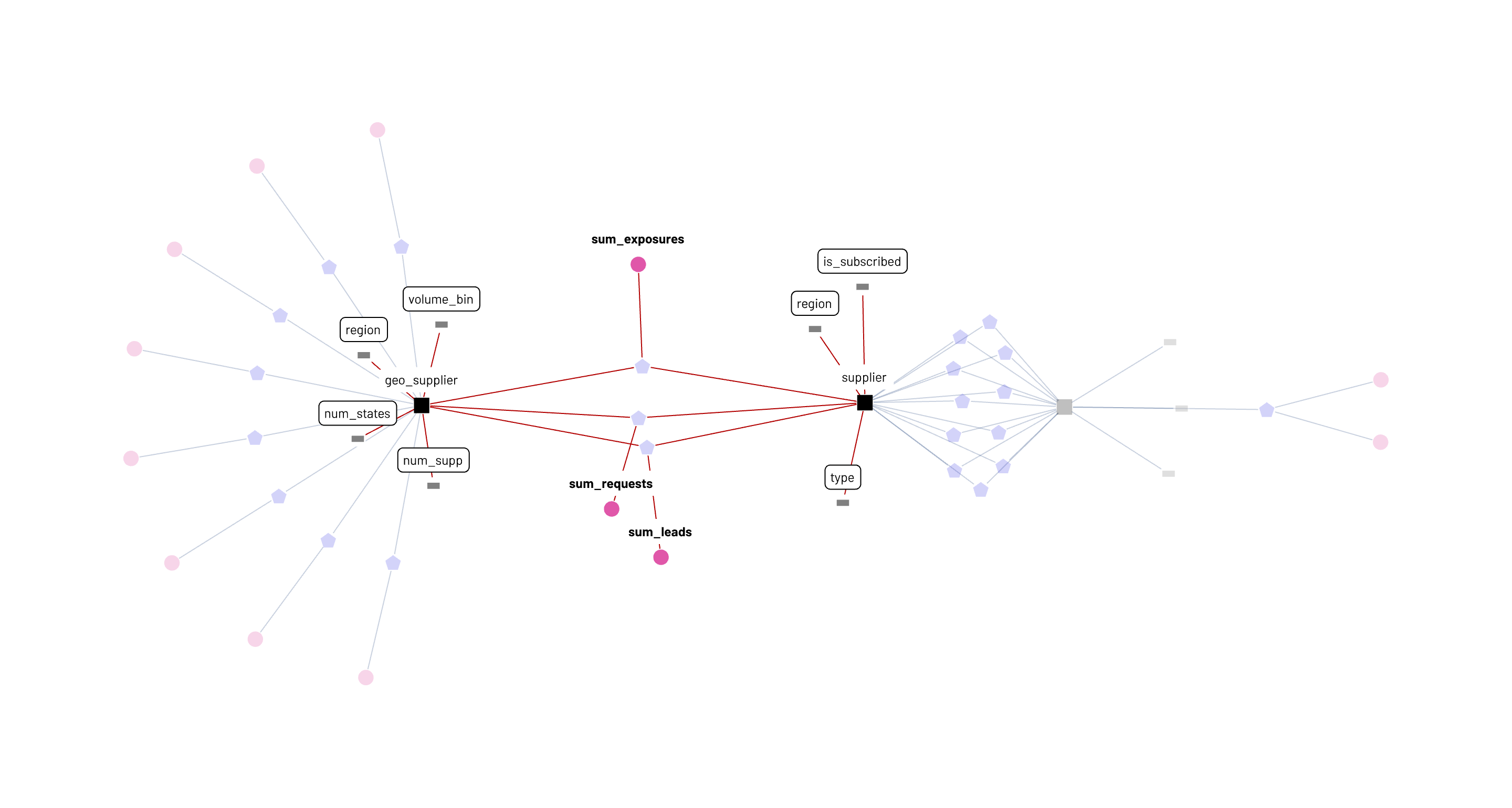
How else can a graph like this help us reason about segmentation? Let’s take another example: a meal kit distributor selling to consumers or users in the graph. If you look at a metric like revenue, it is defined on both users and recipe entities, and based on the existing entity relationships, one can traverse from recipes to suppliers, reach the attributes segment and territory, and pull that in to analyze revenue by. This graph makes it easy to fathom what’s computationally possible from the data infrastructure. In the same graph, a metric like new_customers is defined such that only the user entity’s attributes are available for analysis. This could spark a discussion after which a team member could derive new attributes based on the first recipe consumed by the user, and add that to the system.
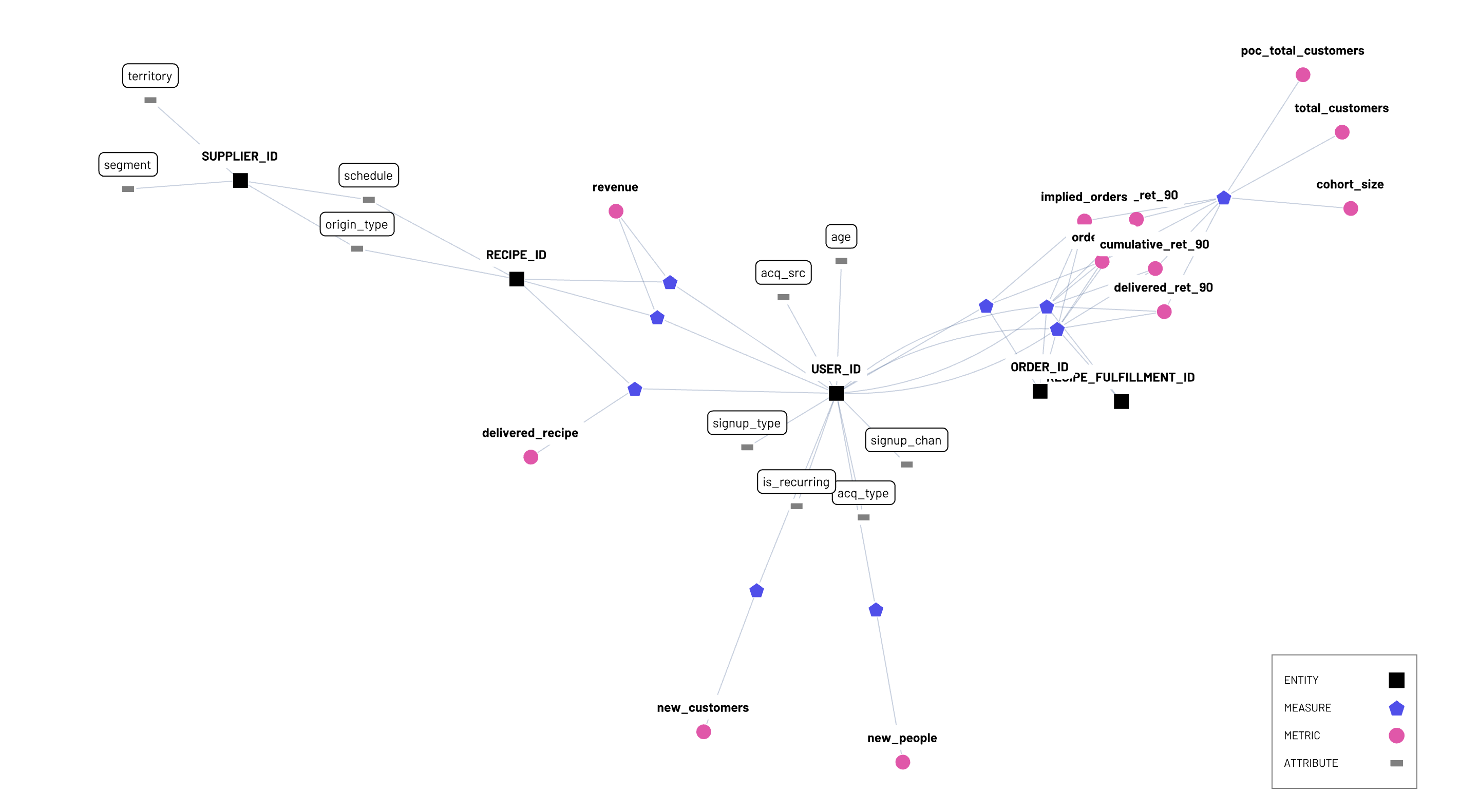
Beyond the ability to drive such collaborative workflows in an org, software can now operate on this graph and as an example, automatically run a segment root cause when revenue goes down unexpectedly - and pin-point which segment and why this happened with just a few clicks. When organizations invest in rich attribution and segmentation, and applications can start exploiting the business semantic relationships via a graph like this, we are looking at the next wave of the data revolution.