As organizations mature in their data journey, deep analytics work is de-prioritized in favor of data science/ML as the latter is seen as the logical evolution in the data journey. There is so much data ROI left on the table when making this choice - so in this post, I want to outline key markers for an analytics practice.
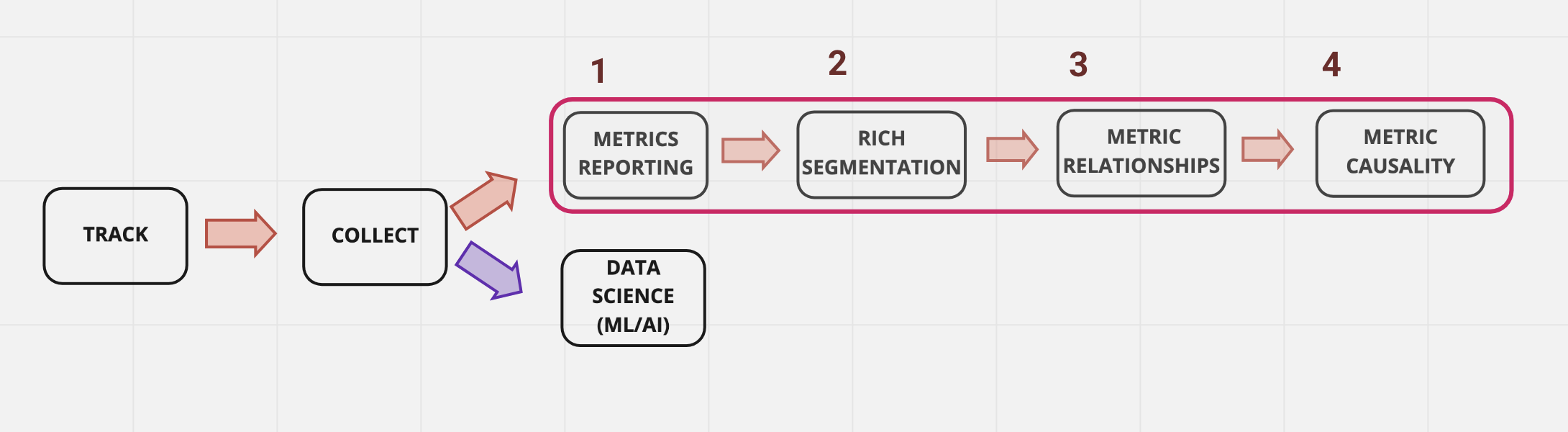
Step 1 naturally starts with metric definitions and reporting. Usually, this step is coupled with default ways to cut or “breakout” the metric. If the metric is conversion rate of signups to customers, you want to cut it by signup channels. The breadth and depth to which metrics can be cut, sliced and diced is such a strong predictor of a data-centric culture that the richness of available segments is a key evolutionary step 2.
As an example for a consumer facing organization, if you are measuring retention on the user entity, you need to not only analyze segment variations across the source channels but model user attributes like their product or consumption experience, their operational experience, their usage of promotions, their demographics, and qualitative feedback data. These will provide directly actionable tactics or indirect insights to shape product strategy. Similarly, there is a rich set of account and usage attributes to analyze metrics within a B2B product company. In fact, any domain across any industry has its own rich tapestry of entities, metrics and attributes (which represent segments).
When metrics are accompanied by a rich understanding of distributions and entity segments, you are in step 2 of the analytics journey. In all the recent buzz around metrics layers, metric stores, and so on, the attributes that represent various entity segments has not been given its due.
Step 3 of the journey is your ability to understand relationships between multiple metrics, and building on step 2, the relationship between segments and multiple metrics. Without this, you will be faced with metrics bloat and sprawl, and struggle to see the forest for the trees. You may end up in the classic “analysis paralysis” state that business leaders are wont to quote in meetings. In this phase, you “reduce” metrics complexity. Then, finally the evolutionary journey culminates when you consistently seek the holy grail of causality - which interventions, and which segments causally impact the desired set of metrics. While causality is always in the background during analytics work, making it an explicit part of the work and dialogue is the hallmark of a data-centric organization.
The reality today is most organizations first build a set of key reports with default segments and either stagnate or they end up creating a sprawl of metric and attribute cuts drowning the organization in noise. The next step in this journey is not automatically machine learning - there is so much data ROI on the table if you invest in rich segmentation, deeply understand the connections between metrics, and explore underlying causality.
The big picture is in the little details.